Market and Impact of generative AI: 9 Uses Cases
Artificial intelligence is expected to run 75% of businesses by 2050. This makes sense considering AI's ability to automate repetitive work and make other processes more cost-effective and efficient. However, because it does not weary or become bored easily, it has been demonstrated to produce greater quality outputs than human-created alternatives. In fact, many fields nowadays rely substantially on AI algorithms, even if the general public is unaware of it! Generative AI is an artificial intelligence branch that generates new and unique ideas. It is a type of machine learning, but it differs from others in that it can create whole new works that are indistinguishable from human efforts.
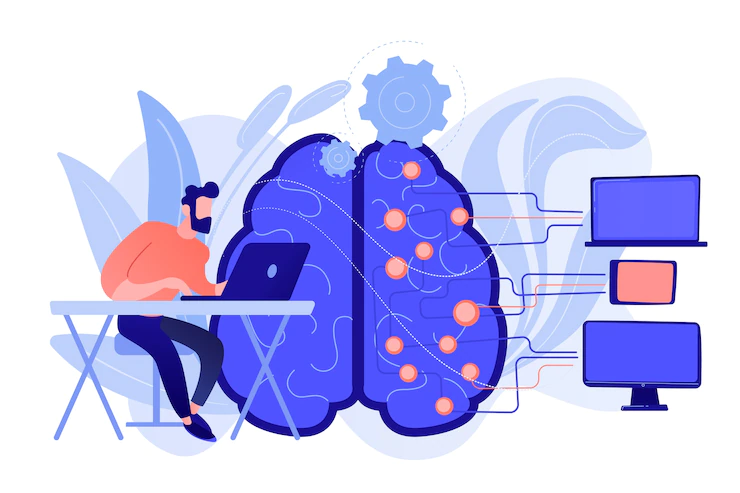
In this blog, we’ll have a deep look at the uses-cases of generative AI in creative industries such as music and art as well as how these technologies are being used by different businesses Nowadays!
What is Generative AI:
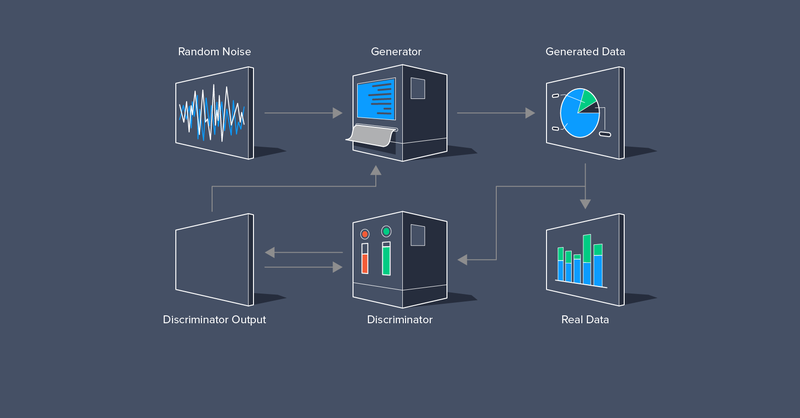
Machines can now create artificial yet original content thanks to generative AI, which employs the algorithm of Artificial intelligence and machine learning. The system does this by using already-existing text files, audio files, image files, etc. The ultimate result is completely new information that deceives the consumer into thinking it is authentic. With generative AI, computers can recognize the underlying pattern in the input given and generate material that is comparable to it. For this, a variety of methods are employed, including Transformers (GPT-3, LaMDA, etc) and Generative Adversarial Networks (GANs). A few of the main processes include generating new content from source data, and distinguishing, and determining whether created data is closest to the original.
The advantages of generative AI are as follows:
- Results of higher quality; produced by self-learning from various data sets
- Reduces risks connected with projects, strengthens devices with less biased machine learning models, and enables depth reduction without sensors
- In both real-world and virtual contexts, robots are more adept at understanding abstract theories.
Important Stats regarding Generative AI:
- According to Gartner, 71% of respondents felt the ROI of intelligent automation is strong within their enterprises.
- By 2025, generative AI will account for 10% of all data produced.
- Between 2020 and 2027, the predicted annual growth rate for AI is 33.2%.
- By 2030, AI is predicted to enhance global GDP by $15.7 trillion, or 26%.
Generative AI in The Digital Transformation Era:
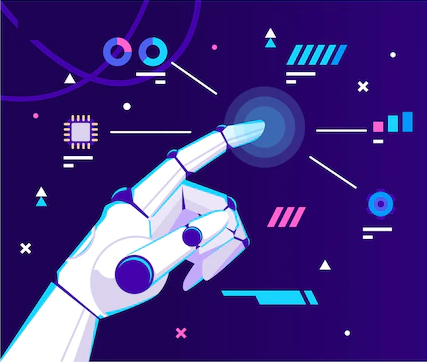
The rapid adoption of artificial intelligence (AI) technology has increased the demand for processes that properly exploit the field's expanding potential. Businesses employ AI technology to diversify working habits and make improvements to continually changing processes as a major component of digital transformation.
The ability of an organization to quickly implement AI technologies contributes to digital transformation in the four essential aspects such as technology, activities, goals, and boundaries. These characteristics aid in the AI framework's preparation and allow for a better theorization of the roles AI will play in the future of digital transformation.
Generative AI will be used more frequently in the future as a tool for digital transformation. This is because generative AI may help organizations seize new opportunities by giving them access to previously untapped data sets that can be utilized to enhance existing procedures.
To stay competitive and sustain a meaningful edge in the market, digital transformation is crucial. Businesses will be able to create new chances for themselves that would otherwise be unavailable with the aid of generative AI. This is because generative AI may help organizations seize new opportunities by giving them access to previously untapped data sets that can be utilized to enhance existing procedures.
Generative AI Techniques:
- Generative Adversarial Networks
A general adversarial network (GAN) is a machine learning system that pits two neural networks against each other. A training set is provided, which enables AIs to generate fresh data with the same statistics as the training set.
- Autoencoders
Data is automatically encoded with the use of autoencoders, which consist of an encoder and a decoder. Unsupervised artificial neural networks' autoencoders memorize and swiftly encode data so that it can be later decoded and reassembled.
Generative AI Market Landscape:
The diagram below explains the platform layer that will power each category as well as the various sorts of apps that will be built on top.
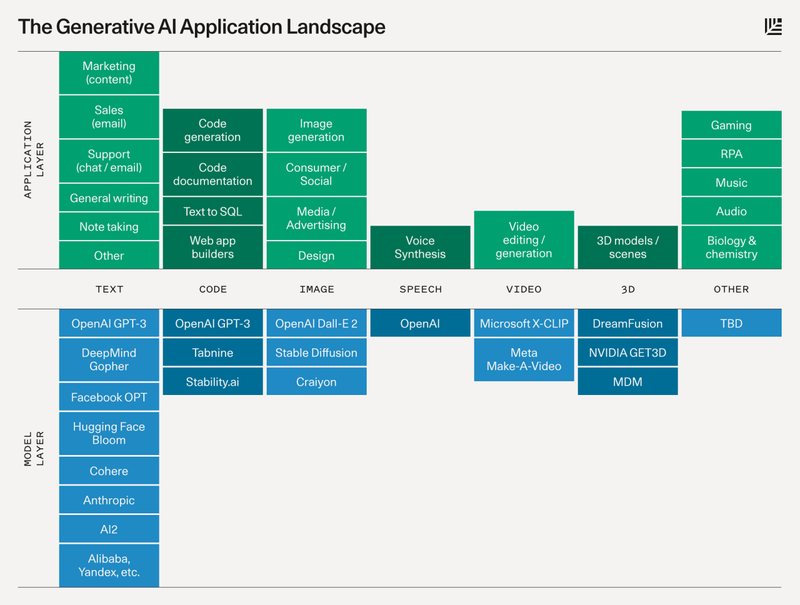
Generative AI Use Cases
- Generative AI in Movie Industry:
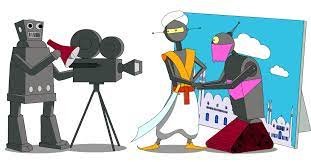
Many vintage movies and classic Disney cartoons are treasures of global culture, but their quality frequently falls short of the expectations of modern times. By generating 60 frames per second as opposed to the typical 23 frames per second, removing noise, and turning black-and-white footage into color, generative AI can upscale them to 4K resolution and even higher.
- Generative AI Animated Model Creation
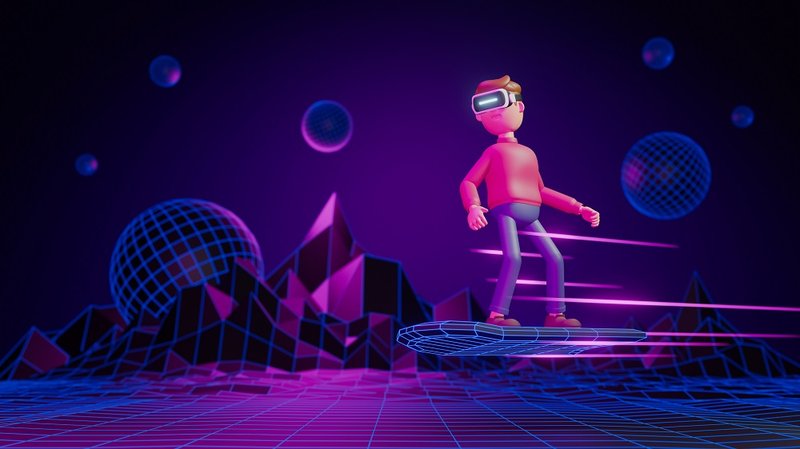
The second area of entertainment that relies on moving pictures, along with the film, is the video game business, and generative AI can aid there too. When AI algorithms create the 3D models used in video games, the work of software developers can be significantly reduced, as well as the time it takes to construct a new piece of software. Such models may be entirely original or derived from previously input 2D photos. Additionally, 2D images can be produced by technology and used in particular game and animation genres, like anime.
- Generative AI in Music World
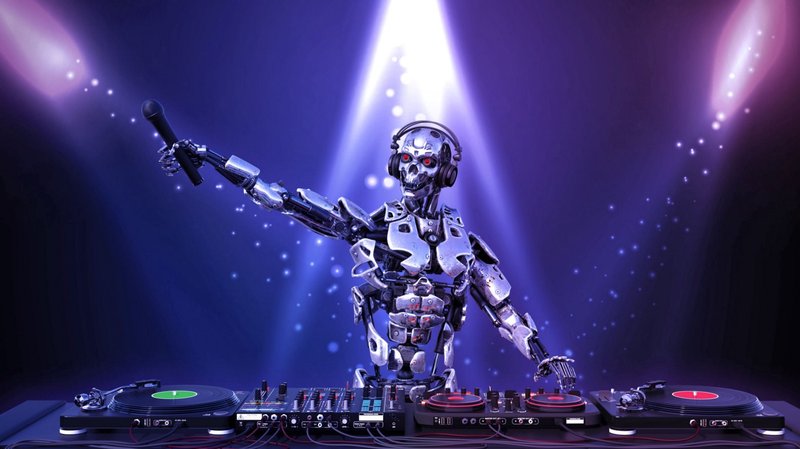
Through the development of neural networks that attempt to replicate and mimic the human brain, generative AI is rethinking the convergence between music and software. Like the human brain, neural networks are able to learn complicated patterns through repetition. The complexity of neural networks is increasing at a tremendous rate, making them more difficult for humans to comprehend.
In 2016, Google's Magenta unit produced the first AI song, and it has subsequently innovated at a record rate. The development of new genres is the main shift that Magenta foresees in the influence of generative AIs on music. Research is being done to examine the effects of combining two or more genres, which will allow AI to play a larger role as a co-creator rather than a tool.
- Generative AI in NFT Creation
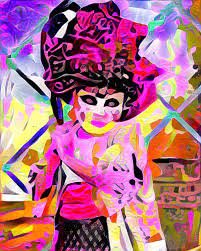
In today's digitally-driven world, NFT (Non-fungible Tokens) and Meta Verse are all the craze and the biggest trend whose sales reached $25 billion last year. NFT art is prominent in the niche, with cartoons, memes, and paintings dominating. Generative AI tools are unrivaled in their ability to create such art pieces that can bring in large sums of money for their creators.
- Generative AI in product design and Object Synthesis
It's important to note that generative AI can design and even produce actual objects like machine parts and household items now that more people are aware of 3D printing. Tools that use AI to carry out or support the design of physical objects include AutoCAD and SOL75.
- Generative AI in Photo Editing
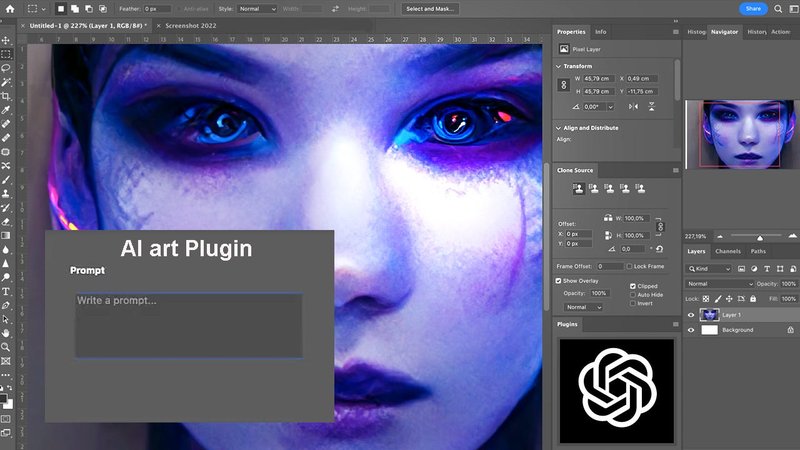
Static photos can be more effectively manipulated using techniques that are used with dynamic ones.
Converting images into images: Poor-quality photos can be improved in resolution, black-and-white photos can be colored, a daytime photo can be changed into nighttime, any photo can be turned into an artistic painting, and satellite photos may be changed into Google maps images.
Analyzing photos of human faces: Generative AI has a wide range of potential applications. It allows you to construct front-on photos from images where a person's face is photographed from an unusual angle, create images of the same person as they age, and even convert real photographs into cartoonists, 3D characters, and emojis. It also allows you to create photos of things and scenes that don't actually exist.
Text-to-picture conversion: You can type text describing a straightforward object (such as a flower or animal) to get a series of photographs that correspond to the description. More complex alternatives will be added to the list of created possibilities as technology advances.
Sketch-to-picture conversion: You can get a realistic picture image of something using a semantic image. This use case is used in the fields of architecture and design (inside and outside of homes and apartments), the fashion industry (sketches of clothing and accessories), the automobile sector (new models of vehicles and trailers), show business (stage scenery), and many other fields.
- Generative AI-based Security services at Airports
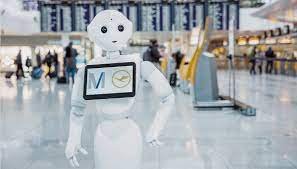
The face verification and identification of people who are missing wanted and whose looks may have changed is a more serious use case. In this scenario, generative AI identification methods won't be hampered by hair color changes, mustache or beard growth, or any other facial expressions captured by the camera.
- Generative AI in HealthCare
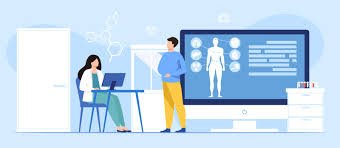
Semantic Image-to-Photo Translation: This technique transforms sketches or images with semantic content into realistic photographs. For instance, diagnosis may be considerably more precise if X-ray or any CT scan images could be transformed into real images.
- Generative AI Sentiment Analysis
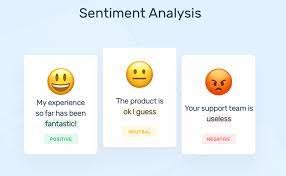
The use of generative AI can be quite beneficial for the retail and commercial sectors as well. People express feelings and rate both the product they purchased and the services the sales organization offered while dealing with items. AI algorithms can be trained to examine user-generated texts, speech samples, and facial expressions that provide insight into how customers feel about a product.
Other generative AI techniques can track the web activity of internet users and evaluate user data to determine how effective a marketing campaign or an advertisement was overall. Such information can also be used to identify different customer segments and create focused marketing campaigns, increasing the likelihood of upselling and cross-selling.
Conclusion:
By self-learning from every set of data, generative AI ensures the production of outputs of a higher caliber. This enables robots to understand, assess, and comprehend novel, abstract, ideational, and conceptual principles.
Unsupervised learning enables AI to develop more quickly and flexible transferable skills that improve the efficiency, effectiveness, and speed of human work while requiring less staff training. The foundation for applications in important industries like defense, security, and healthcare is being laid by generative AI. Technology can be adjusted and integrated into more complex applications as it advances and innovates.
In addition to providing organizations with substantially speedier and less expensive access to picture generation, film restoration, and the construction of 3D or SaaS models or settings, generative AI models are a viable replacement for some of the older, outmoded technology.